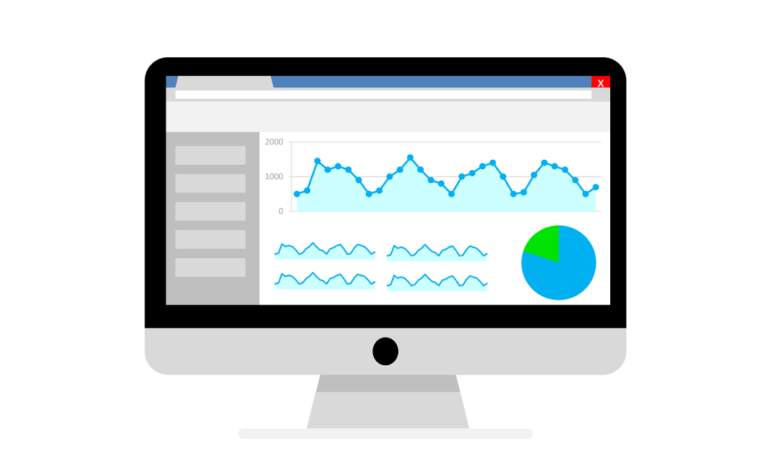
The Revolution of AI In Loan Underwriting
In the present-day context, the advent of powerful algorithms and artificial intelligence, or AI, is affecting all tiers of business around the work across world with the same force altering the dimensions of the financial sector as well.
Machine learning for the underwriting of loan origination is perhaps one of the most groundbreaking approaches to the use of AI in the financing industry. This article explores how AI revolutionizes loan underwriting by streamlining data analysis, enhancing decision-making capabilities, and significantly reducing turnaround times for loan approvals. Analyze financial information to improve decision-making.
Evolution Of Loan Underwriting
In the past, underwriting of loans was a very cumbersome affair, which involved manual work where loan officers diligently went through the applicant’s details and the documentation provided, credit history, and risk profiling. This manual effort was not just timely but also brought the challenge of biases and inaccuracy from one decision-maker to another. Additionally, the sheer number of loan applications that had to be considered required an improved way of sorting them out.
Historical Context
The history of loan underwriting can be traced back to the early 1900s which saw the formation of long-term lending practices. In the early days of underwriting, there was a strong reliance on word-of-mouth referrals, as well as crude ways of looking at the borrower’s repayment ability. The banking industry advanced and therefore the method of how loan underwriting was completed also became increasingly complex.
In the mid of the twentieth century, more systematic credit scores followed by consistent credit reports gave a new dimension to credit policies. However, even with these advancements, the process remained as labor-intensive and slow and this created some bottlenecks in lending.
Challenges Of Traditional Underwriting
The traditional loan underwriting process involves several steps: applying and verifying the applicant’s details, financial position, and creditworthiness, and concluding granting loans. Every stage involves considerable efforts to analyze the working processes, as well as time and money consumption.
Some of the key challenges associated with this approach include:
Human Error: Any type of manual operation in the data process and data checking exposes the transactions to an increased risk of producing wrong results that may lead to wrong lending opportunities being given out.
Inconsistency: Each underwriter may have a different perception of some information that is used leading to varying decisions.
Time-Consuming: The diagnosis of the loan applications is manual hence leading to a very long time being taken to process and approve the loans.
Scalability: This is because as the volumes of loan applications increase, traditional approaches of credit underwriting fail to cope with the increasing demand hence resulting in increased inefficiencies and time delay.
AI-Powered Data Analysis
The AI algorithms are particularly good at analyzing large volumes of information within a short period while at the same time increasing accuracy. In loan underwriting, AI can integrate and evaluate multiple loan data to essentially evaluate borrowers’ ability to repay the loans and the risk that the potential borrower brings. These data sources may include
Credit Scores & Histories: Credit score provides credit history needed by the AI algorithms for determining future behavior towards payment.
Income and Employment Data: Employment details and income sources’ verification is a way of enhancing the electronic underwriting process thus establishing borrowers’ stability.
Bank Statements & Financial Transactions: The transactional data contains key pieces of information regarding income and spending habits and AI can analyze the patterns to ensure its authenticity.
Alternative Data Sources: This adds the social media profiles, utility bill payments, and rental payment history to the list of data that are analyzed; not only the credit metrics.
Advanced-Data Processing Capabilities
Traditional information systems cannot handle the workflow of analyzing data from various sources at the same time and therefore cannot present a complete picture of an applicant’s financial situation in the event of a loan.
These are the important information that is commonly used such as credit scores, employment history, and other innovative information which include for instance the history of the activity on the social media pages, transactions, and other details.
AI being a machine learning technology can process large volumes of data at a go and even present hidden patterns for underwriters to ascertain. It provides more capabilities of data processing to make further qualified and characteristic credit risk evaluations.
Benefits Of AI-Powered Data Analysis
Accuracy: Automating the process of data assessment with the help of AI algorithms decreases the chances of mistakes during the underwriting process.
Efficiency: AI is capable of analyzing big data in the shortest time possible, this means that time spent on the underwriting process is highly minimized.
Comprehensive Assessment: Because AI utilizes numerous variables in the decision-making process and is not limited to the data from the applicant’s formal credit report, the outcome results in a better evaluation of the overall applicant rating.
Enhanced Decision-Making Capabilities
The AI applied or used in loan underwriting entails a machine learning feature that enhances its ability to learn from the inputted data and get better over time. These models can:
Risk Assessment: AI models evaluate general credit risk and specific risks related to the applicants for loans, default probabilities, and fraud.
Loan Pricing: Accordingly, using risk evaluations, AI assists in defining interest rates, loan volume, and conditions acceptable for borrowers’ risk levels and the current market.
Approval Process: This way, conventional decision-making procedures help to accelerate the approval of applicants with low risk and improve the organization of the work of the loan processing department.
Machine Learning & Predictive Analytics
As demonstrated earlier, underwriting is an essential part of AI technologies, and machine learning models are its cornerstone. These models are based on past data as current data inputs refine well-being and improve the forecast’s predictive capacity.
Using data mining techniques, patterns that reflect the potential of a loan to be in the defaulted category are determined by machine learning algorithms, thus increasing the chances of the lender making the right decisions. Credit scoring also has significant implications for loan pricing and loan contractual terms hence the evaluation of the credit risk for each loan.
Minimizing Bias In Decision-Making
AI decision-making is one of the most beneficial applications as it eradicates all possibility of bias. This process is naturally bound to socialstinian biases typical for people at that time regarding particular clients or groups of people based on their ethnicity, gender, or social status.
AI, on the other hand, does look at the deterministic data and avoids any subjectivity that might put a barrier between a customer and his/her loan. In this way, AI also makes lending fairer by avoiding the situation when mortgage applications from members of some groups would be approved significantly more often than others.
Reducing Turnaround Times
Another massive advantage of using AI in loan underwriting is that it can fasten the approval time for loans massively. Old methodologies in underwriting were slow and also time-consuming since officers had to look at the documents and make their own decisions on the loans to grant. AI automation accelerates this process by:
Real-Time Data Processing: When it comes to the verification of the information provided by the applicants and documents, AI processes data and analyzes them in real time.
Automated Document Verification: OCR and NLP utilizing AI to draw out appropriate data from the documents help to minimize the critical review.
Predictive Analytics: Here, AI optimizes the flow of loan decisions without any influence on the quality of the given decision, as these outcomes depend on historical experience and the current state of the market environment.
Impact On Customer Satisfaction
The fact that the organization has been able to reduce its turnaround time is a direct implication that there has been an improvement in the quality of customer satisfaction. Loan seekers do not have to wait for days to receive responses on their loan approval as is the case in the traditional methods.
Rather they get a response within hours or sometimes it is within minutes thus enriching their experience. This is very advantageous given the nature of the lending markets where borrowers may apply with several lenders and go with the one who responds first.
Operational Efficiency For Lenders
Reduced turnaround time has a benefit to lending institutions since it enhances the operations of the undertaking. Automation made possible by AI enables firms in lending to approve a large number of applications without having to hire more staff.
This efficiency gain also helps to reduce the operational cost while at the same time assisting the lenders to exploit market opportunities as and when they arise. AI makes it easier for lenders to continue providing credit due to slashing the time taken in underwriting.
Challenges & Considerations
While AI offers significant advantages in loan underwriting, there are several challenges and considerations to address:
Data Privacy and Security: Borrower data involve several aspects of privacy thus, it calls for stringent security measures and conformity to laws on data privacy.
Interpretability of AI Models: The obligation to explain how an AI decision was made to achieve compliance with regulations, as well as to maintain the population’s trust.
Integration with Existing Systems: AI systems integration capabilities that allow them to deploy such systems as integrated components of existing IT architectures and protocols for boosting effectiveness.
Addressing Data Privacy & Security Concerns
Financial information is usually more sensitive, so its protection must be beyond reproach. Lenders need to ensure that borrowers’ details do not fall into the wrong hands and adhere to the GDPR and CCPA among other legal requirements. Also, the usage of various codes and encryption methods and the proper storage of the data may reduce the threat of theft and hacking attempts.
Ensuring Transparency & Explainability
AI for loan underwriting has certain concerns, including Explainable AI, which focuses on being able to explain why a particular decision has been made. The legal bodies demand that credit decisions are justified so that there is fairness and efficiency.
To achieve this, it is essential to build MI-L models that autistically can explain their recommendations. Besides, it assists in the fulfillment of legal demands regarding disclosure, which is important for generating trust from borrowers, especially when they need to know why they have been approved a loan or rejected.
Integrating AI With Legacy Systems
Two, AI systems must seamlessly integrate with a company’s existing IT structures to achieve optimal results. Most of the lending institutions integrate the use of outdated systems that may not be compatible with modern AI technologies.
Solving this problem implies system integration as a strategic activity with the application of middleware and API for transferring the necessary information between legacy systems and AI software. Therefore, by proper integration of the systems, the lenders will be able to achieve the envisioned benefits of AI in underwriting.
Future Directions
AI in loan underwriting, therefore, has a promising future because it will be subjected to additional improvements in the future. Possible future trends consist of more advanced applications of forecasting, expansion of the use of various types of external data, and progress in the related problem of artificial intelligence and its regulation.
Enhanced Predictive Analytics
In the future, when the AI innovation will continue to outweigh, predict analysis will be finer than the existing one. Superior learning models of the future will be able to allow for greater or improved data analyses to make better or more accurate risk evaluations as well as credit decisions. Both these models will also incorporate instantaneous market data as well as other economic parameters, thus offering credit risk reviews to lenders that are timely and elaborate.
Broader Integration of Alternative Data Sources
The implication of the advanced application of AI underwriting for future loan processes will incorporate more data options. Thus, about the peculiarities of modern business, the financial performance data together with the financial performance ratios derived from them will be supplemented with the data received from sources that have not been used earlier, for example, data from social networks, observing the credit holder’s behavior on the Internet, or biometric data. With the integration of such an array of data sources, AI models can better perceive the borrowers’ overall picture and provide equal and rightful creditworthiness to deserving individuals.
Advancements In AI Ethics & Governance
With the increased adoption of AI in loan underwriting future developments would emphasize the issues of AI ethical and governing policies. The accuracy of the models will not be very useful if it is not accompanied by fairness, transparency as well as accountability of the AI models. This comprises setting standards on how AI can be used, creating frameworks for the supervision of AI-made decisions, and culture transformation towards safe AI.
Excerpt
In the present world of technology, innovations in artificial intelligence (AI) are revolutionizing institutions, specifically the finance domain. This article explores how AI is now being applied in the processing of loan underwriting and its effect on data processing decision-making, and times taken. Starting from the transition from the conventional underwriting process to the implementation of AI in data processing, this article examines how AI boosts precision, productivity, and consumer happiness.
Also, we talk about the issues of data access, privacy, openness, and interoperability with other systems. These are the areas of nonmedical AI development for the future, improved forecasting methods, increased data scope, and improvements in AI moral standards. Read more about these innovations on Vortex Pedia, the source of perfect guest posts on future trends.